Top 6 Data and Analytics Trends that will change your business
4th December 2019
The role of data and analytics in digital transformation is becoming more strategic. Due to the increasing volume and complexity of data that organisations hold, the types of analysis available to data scientists, and the need for increasingly quick analysis of vast sets of data in an increasingly connected world, organisations will need to adapt in order to succeed. Our experts break down the data and analytics trends that you need to know.
With increasing complexity in analytics and in ML and AI comes new and evolving challenges that organisations will need to meet in order to successfully innovate for a competitive advantage, regardless of industry or scale.
Below are the six data and analytics trends that will change your business in 2020 and beyond.
- Augmented Analytics
- Augmented Data Management
- ‘Always On’ Analytics
- NLP and Conversational Analytics
- Graph Analytics
- Explainable AI
Trend One: Augmented Analytics
One of the most important data and analytics trends to understand is augmented analytics. Augmented analytics as defined by Gartner ‘enables machine learning and AI-assisted data preparation, insight generation, and insight explanation to augment how business users and analysts explore and analyse data in analytics and BI platforms.’
As data collection volume and complexity increases, decision-makers access to an abundance of data – and confusion. It can be difficult to identify what data is most important, and what actions to take (or avoid) based on scale.
Larger and more-varied dataset combinations also bring additional challenges – more and more data to investigate, analyse, and govern. These tasks are becoming increasingly difficult to manage efficiently using current manual approaches.
Augmented analytics automates parts of discovering and unearthing the most important insights from the data to optimise decision-making. Using ML to automate parts of the process increases the speed with which insights and the relationships between datasets can be discovered and presented to non-technical business users.
An additional business benefit of this trend is that, while it will require an increased focus on organisation-wide data literacy, augmented analytics enables business users to unearth relevant insights independently, freeing data scientists for more high-value tasks. Putting in place processes to promote cross-department collaboration while leveraging the capabilities of augmented analytics will be critical to success.
Trend Two: Augmented Data Management
The volume and diversity of data being generated every day is growing at a rapid pace. IBM estimates that 2.3 trillion gigabytes of data are created each day. At the same time, it is being generated from many various trusted and untrusted data sources. The volume and diversity of data being generated has established the need to automate data management tasks that respond to governance policies, rules and processes.
Similar to how ML and AI capabilities are transforming organisations’ analytics capabilities, ML capabilities and AI engines are being developed to make self-configuring and self-tuning processes. These processes are automating many of the manual tasks previously dedicated to highly skilled data scientists and allowing users with less technical skills to be more autonomous when using data. Gartner estimates that by 2023, AI-enabled automation in data management will reduce the need for IT specialists by 20%.
An overview of the impact of automation on the data management capabilities of an organisation:
- Augmenting or automating data engineering tasks
- Avoiding potential errors related to human interaction with data
- Automating the tracking and reporting of errors in datasets
- Increasing the capability to use data
Trend Three: ‘Always On’ Analytics
‘Always On’ Analytics combines analytics with transactional real-time business processes. It leverages augmented analytics, event stream processing, optimization, business rule management and ML.
‘Always On’ Analytics can be broken down into three areas:
- Situation awareness is real-time or near-real-time, based on continuous (always on) ingestion of streaming data
- Proactive behaviour means that the system can push alerts, update dashboards or trigger automatic responses when it detects a situation that requires attention (it’s not just reactive, waiting for a person or application to inquire or pull information).
- Prescribing behaviour means that the system tells you what to do. It provides decision support for a human decisions or decision automation for lights-out processes (in other words, it’s not just notifying you of what’s happening and making you figure out an appropriate response).
‘Always On’ analytics improves the accuracy and confidence of critical operational decisions because it incorporates up-to-date and trusted data. It is relevant to real-time and near-real-time decisions where there is a benefit to having an understanding of the current situation (or events in the past few seconds or minutes).
Systems are able to process high volumes of data quickly, shielding people from overload. They are able to apply rules and optimisation logic to evaluate far more options than a person could consider in the available time.
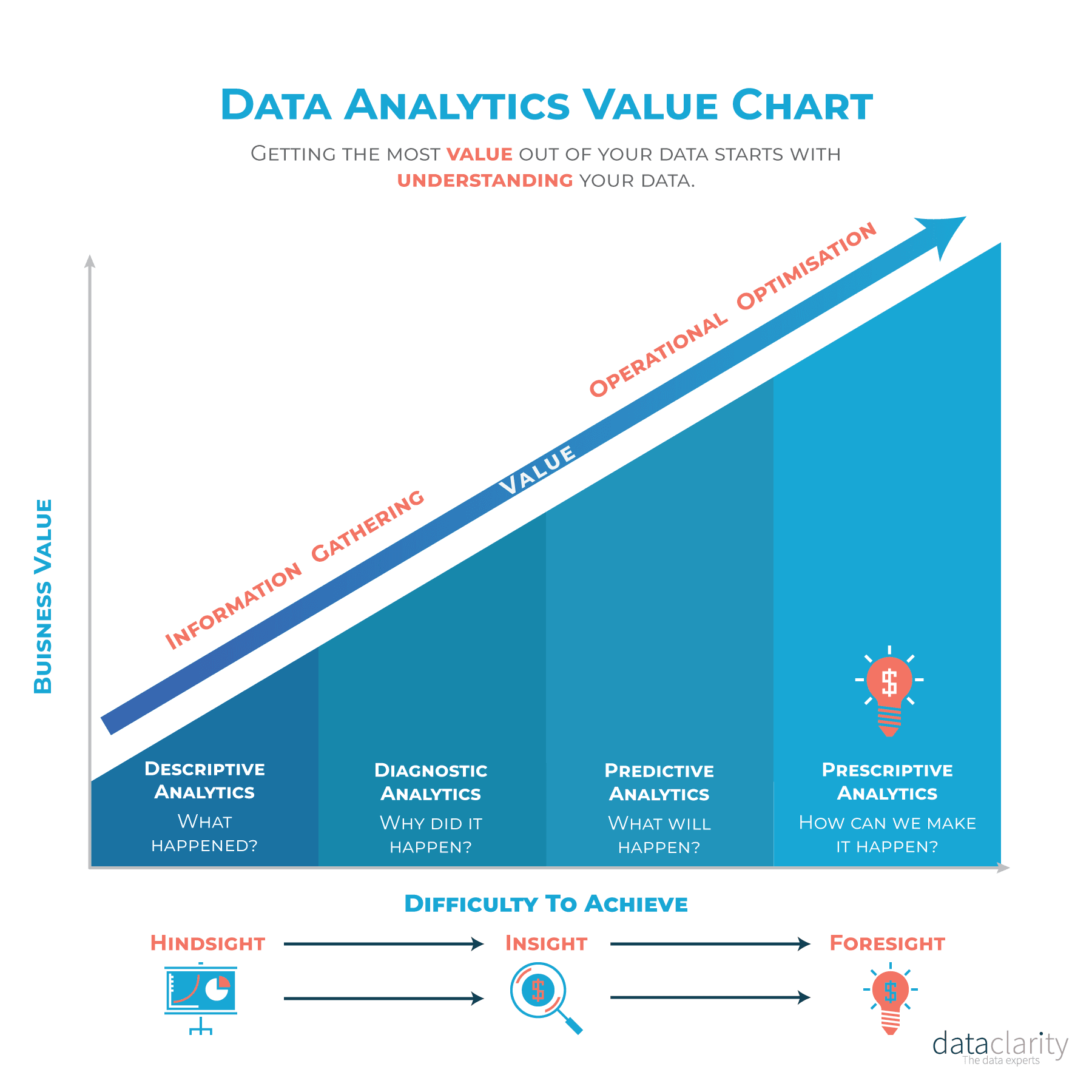
Trend Four: NLP and Conversational Analytics
Traditionally, access to analytics content from BI platforms has mostly been limited to power users, business analysts and specialist data scientists with varying degrees of analytical and technical skills. NLP (Natural Language Processing) provides users with a simpler method of interrogating data without needing technical skill.
Just as search interfaces like Google made the internet accessible to everyday users and consumers, search and natural language query (NLQ) approaches to asking questions of data enable mainstream business users to access data analytics.
As more organisations undergo digital transformation, analytics becomes a critical decision-making tool and legitimate competitive advantage. Expanding access to insights from analytics to all workers will be key to driving transformation and data culture.
Any user can ask questions using text or voice with increasingly complex questions and responses. NLP is increasingly an interface to querying and interacting with autogenerated insights from augmented analytics.
The combination of NLP with augmented analytics — including automatic insight generation — allows users to rapidly find the most important and actionable insights via conversational analytics with natural language generation.
Trend Five: Graph Analytics
Business users increasingly needing the answers to complex questions across various datasets. Complex analysis often requires blending data from multiple sources, multiple business units, and increasingly external data.
Combining sets such as weather, economics, production, personnel, regulations, performance metrics, and others are complicated when it comes to assuring that content and media are relevant and trusted. Analysing and extracting insights from vast and various data sets at scale is not practical, or in some cases possible, using traditional query tools.
Many use cases have graphs as their enabling technology. Graph analytics are typically portrayed via a visualization for business-user consumption. In graph visualization, it is possible to drag one metric inside of another, or remove part of a metric to recombine it with other datasets. Graph analytics consists of models that determine the “connectedness” across data points.
Trend Six: Explainable AI
Explainable AI is the set of capabilities that describes a model, highlights its strengths and weaknesses, predicts its likely behaviour, and identifies any potential errors. As we move towards more augmented analytics including autogenerated insights and models, the ability to explain how these insights and models are generated will become critical to build corporate trust, compliance with various global regulatory bodies, and to manage brand reputation.
Without acceptable explanation, autogenerated insights and models, or black boxes combined with AI bias, can cause concerns about regulation, reputation, accountability and fairness, and lead to distrust in AI solutions.
Explainable AI enables a better adoption of AI by increasing the transparency and trustworthiness of AI solutions and outcomes. Explainable AI also reduces the risks associated with regulatory and reputational accountability for safety and fairness.
Increasingly, these solutions are not only showing data scientists the input and the output of a model, but are also explaining the reasons the system selected particular models and the techniques applied by augmented data science and ML.
Conclusions
To benefit from the volume of data collected every data and to create a pervasive data culture means that you will have to apply data and analytics initiatives more broadly to all parts of the business.
As highlighted in the data and analytics trends we have focused on, increasing data literacy and investing in analytics as a strategic priority aligned to business priorities and outcomes, and pushing it out to all corners of the business, is an imperative. Delivering on most digital business goals and objectives will depend on it.
For more information about the current data and analytics trends in retail technology, download our whitepaper or to discuss how to get the most out of your organisation’s data and how it can boost your current strategy, contact us.